Abstract
Online algorithms gain prominence as the volume of data explodes, among which second-order methods are known for their robustness. While much research focuses on the convergence of stochastic second-order methods, the algorithms’ variability remains underexplored. This paper studies the statistical inference of the estimates generated by an inexact adaptive stochastic Newton method. The algorithm not only allows adaptive stepsize, but also significantly reduces computational costs by inexactly solving the Newton systems with a randomized solver involving sketching techniques. For the designed algorithm, we establish the asymptotic normality of its last iterate and, more importantly, construct a fully online limiting covariance estimator. Our covariance estimator solely utilizes the iterates (with varying weights) from the stochastic Newton algorithm, which outperforms the plug-in estimator in terms of both consistency and computational efficiency. We establish the convergence rate of the weighted covariance estimator and illustrate its superior performance on regression problems.
Publication
A short note is accepted by 2023 NeurIPS Optimization for Machine Learning (OPT) workshop
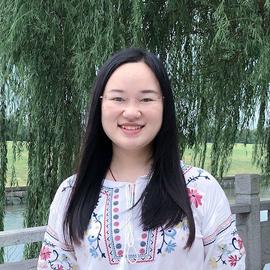
PhD in Statistics (2019-)
Wei Kuang is currently a PhD student in the Statistics department at UChicago, working with Mihai Anitescu (supervisor) and Sen Na on randomized second-order methods, with an emphasis on the uncertainty quantification and statistical inference aspects.
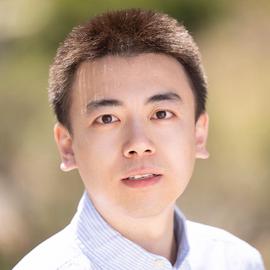
Assistant Professor in ISyE
Sen Na is an Assistant Professor in the School of Industrial and Systems Engineering at Georgia Tech. Prior to joining ISyE, he was a postdoctoral researcher in the statistics department and ICSI at UC Berkeley. His research interests broadly lie in the mathematical foundations of data science, with topics including high-dimensional statistics, graphical models, semiparametric models, optimal control, and large-scale and stochastic nonlinear optimization. He is also interested in applying machine learning methods to biology, neuroscience, and engineering.
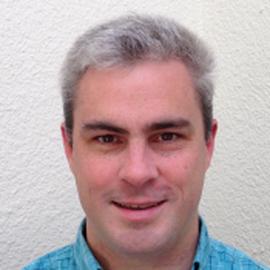
Professor in Statistics and ICSI, Amazon Scholar
Michael Mahoney is a Professor in the Statistics department and ICSI at UC Berkeley. He is also the director of the NSF/TRIPODS-funded Foundations of Data Analysis (FODA) Institute at UC Berkeley. He works on the algorithmic and statistical aspects of modern large-scale data analysis. Much of his recent research has focused on large-scale machine learning, including randomized matrix algorithms and randomized numerical linear algebra.
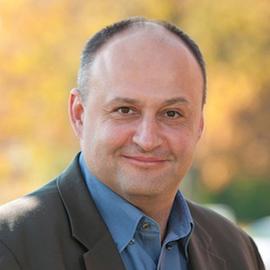
Professor in Statistics and CAM
Mihai Anitescu is a Professor in the Statistics and CAM departments at the University of Chicago, and is also a senior computational mathematician in the Mathematics and Computer Science Division at Argonne. He works on a variety of topics on control, optimization, and computational statistics.