Abstract
We study the performance of stochastic predictive control (SPC) for linear systems with a quadratic performance index and additive and multiplicative uncertainties. Under a finite support assumption, the problem can be cast as a finite-dimensional quadratic program, but the problem becomes quickly intractable as the problem size grows exponentially in the horizon length. SPC aims to compute approximate solutions by solving a sequence of problems with truncated prediction horizons and committing the solution in a receding-horizon fashion. While this approach is widely used in practice, its performance relative to the optimal solution is not well understood. This article reports for the first time a rigorous performance guarantee of SPC: under the standard stabilizability and detectability conditions, the dynamic regret of SPC is exponentially small in the prediction horizon length. Therefore, SPC can achieve near-optimal performance – the expected performance can be made arbitrarily close to the optimal solution – at a substantially reduced computational expense.
Publication
arXiv preprint arXiv:2210.08599
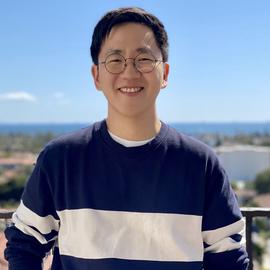
Postdoc in Mathematics and Computer Science Division
Sungho Shin is a postdoc in the Mathematics and Computer Science Division at Argonne National Laboratory. His research interests include model predictive control, optimization algorithms, and their applications to large-scale energy infrastructures (such as natural gas and power networks).
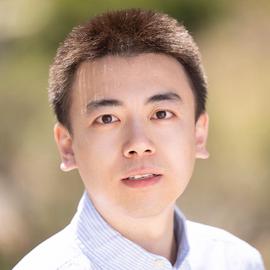
Assistant Professor in ISyE
Sen Na is an Assistant Professor in the School of Industrial and Systems Engineering at Georgia Tech. Prior to joining ISyE, he was a postdoctoral researcher in the statistics department and ICSI at UC Berkeley. His research interests broadly lie in the mathematical foundations of data science, with topics including high-dimensional statistics, graphical models, semiparametric models, optimal control, and large-scale and stochastic nonlinear optimization. He is also interested in applying machine learning methods to biology, neuroscience, and engineering.
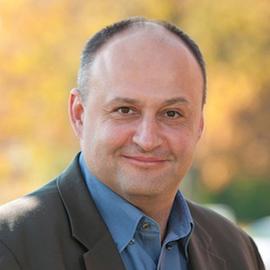
Professor in Statistics and CAM
Mihai Anitescu is a Professor in the Statistics and CAM departments at the University of Chicago, and is also a senior computational mathematician in the Mathematics and Computer Science Division at Argonne. He works on a variety of topics on control, optimization, and computational statistics.