Abstract
We propose a fast temporal decomposition procedure for solving long-horizon nonlinear dynamic programs. The core of the procedure is sequential quadratic programming (SQP) that utilizes a differentiable exact augmented Lagrangian as the merit function. Within each SQP iteration, we approximately solve the Newton system using an overlapping temporal decomposition strategy. We show that the approximate search direction is still a descent direction of the augmented Lagrangian, provided the overlap size and penalty parameters are suitably chosen, which allows us to establish the global convergence. Moreover, we show that a unit stepsize is accepted locally for the approximate search direction, and further establish a uniform, local linear convergence over stages. This local convergence rate matches the rate of the recent Schwarz scheme (Na et al., 2022). However, the Schwarz scheme has to solve nonlinear subproblems to optimality in each iteration, while we only perform a single Newton step instead. Numerical experiments validate our theories and demonstrate the superiority of our method.
Publication
Mathematics of Operations Research
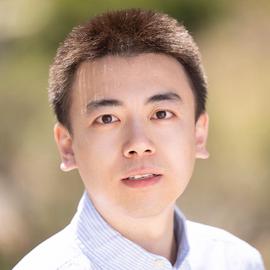
Assistant Professor in ISyE
Sen Na is an Assistant Professor in the School of Industrial and Systems Engineering at Georgia Tech. Prior to joining ISyE, he was a postdoctoral researcher in the statistics department and ICSI at UC Berkeley. His research interests broadly lie in the mathematical foundations of data science, with topics including high-dimensional statistics, graphical models, semiparametric models, optimal control, and large-scale and stochastic nonlinear optimization. He is also interested in applying machine learning methods to biology, neuroscience, and engineering.
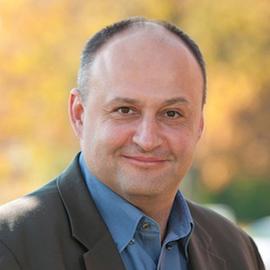
Professor in Statistics and CAM
Mihai Anitescu is a Professor in the Statistics and CAM departments at the University of Chicago, and is also a senior computational mathematician in the Mathematics and Computer Science Division at Argonne. He works on a variety of topics on control, optimization, and computational statistics.
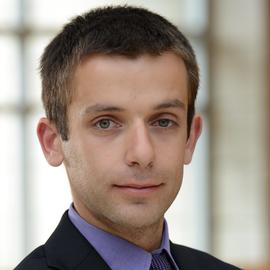
Associate Professor of Econometrics and Statistics
Mladen Kolar is an Associate Professor of Econometrics and Statistics at the University of Chicago Booth School of Business. His research is focused on high-dimensional statistical methods, graphical models, varying-coefficient models and data mining, driven by the need to uncover interesting and scientifically meaningful structures from observational data.